Lukas Gosch
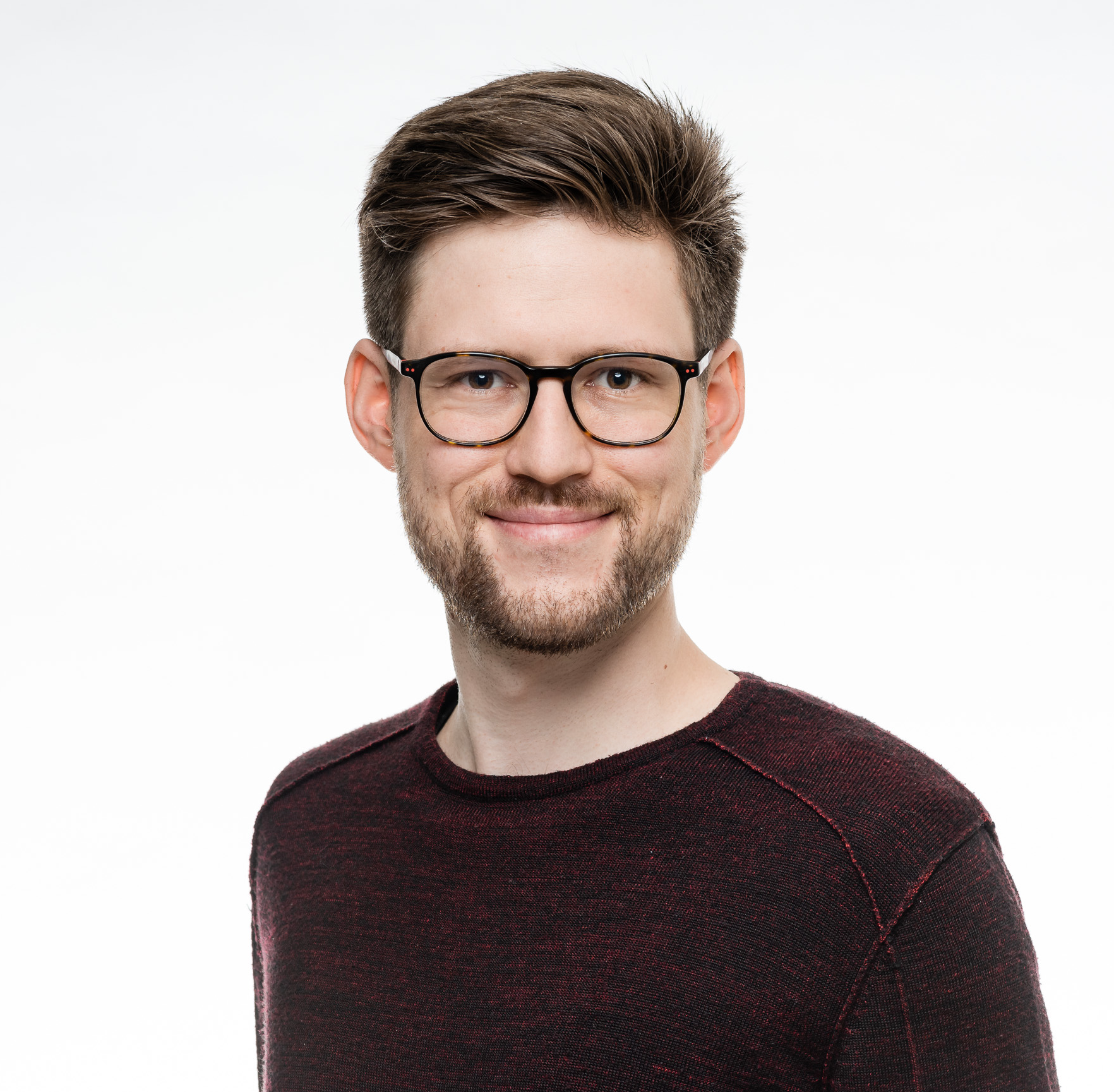
Hello, welcome to my corner of the web!
I am a researcher on machine learning focusing on robust and reliable learning on graphs. I am doing my PhD at TU Munich under the supervision of Prof. Günnemann in the DAML research group and am part of the relAI graduate school. Currently, I am especially interested in provable robustness. Furthermore, I am also interested in theoretical machine learning, high-dimensional statistics and combinatorial optimization.
If you want to contact me, best write me an e-mail: lukas . gosch [at] tum.de. Scroll down to find my other social media appearances.
Quick Link: Resume/CV
news
Oct 1, 2023 |
Our paper Adversarial Training for Graph Neural Networks: Pitfalls, Solutions, and New Directions got accepted at
NeurIPS 2023 ![]() |
---|---|
Jun 1, 2023 |
Our paper Expressivity of Graph Neural Networks Through the Lens of Adversarial Robustness got accepted at
AdvML Frontiers@ICML2023 ![]() |
Feb 1, 2023 |
Our paper Revisiting Robustness in Graph Machine Learning got accepted at ICLR 2023 ![]() |
Dec 9, 2022 | I gave an oral talk today at TSRML@NeurIPS22 about Revisiting Robustness in Graph Machine Learning. |
Nov 1, 2022 |
Our paper Training Differentially Private Graph Neural Networks with Random Walk Sampling got accepted at the TSRML@NeurIPS22 workshop ![]() |
Nov 1, 2022 |
Our paper Revisiting Robustness in Graph Machine Learning got accepted and selected for an oral talk at
TSRML@NeurIPS22 and accepted at ML-Safety@NeurIPS22 ![]() |